Machine learning, a subset of artificial intelligence, has revolutionised numerous industries and become an integral part of modern businesses. With its ability to analyse vast amounts of data and uncover hidden patterns, machine learning has opened up new avenues for growth, efficiency, and innovation. From personalised marketing to risk assessment and process automation, the impact of machine learning is profound. In this article, we will explore the importance of machine learning in business and how it reshapes how organisations operate.
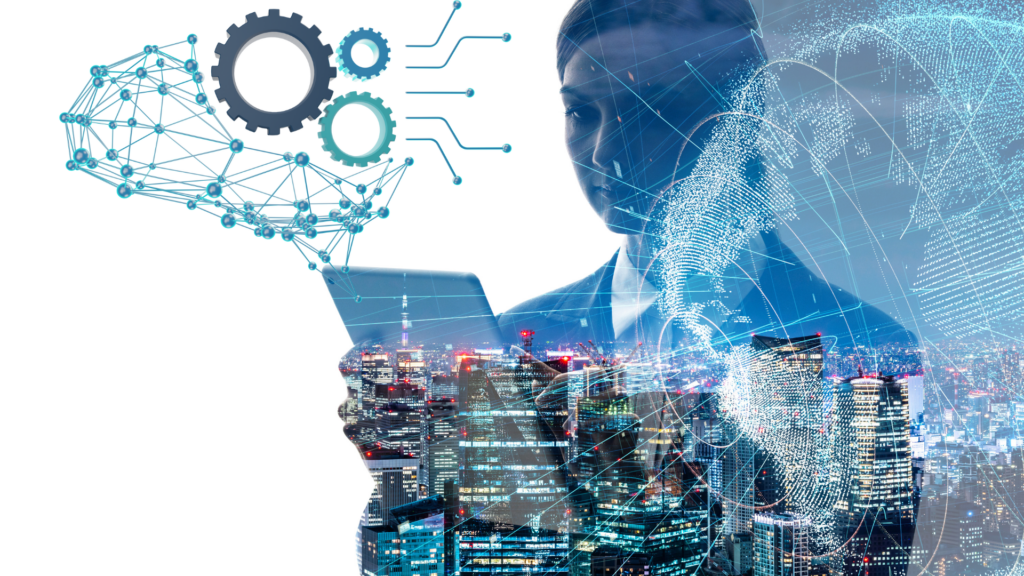
Table of Contents
- The Impact of Machine Learning on Business
- Enhanced Decision-Making Processes
- Improved Customer Experience
- Streamlined Operations and Cost Reduction
- How can you use machine learning in your business?
- The Future of Machine Learning in Business
- Advancements in Natural Language Processing
- Integration of Machine Learning with IoT
- Potential for Autonomous Systems and Robotics
- FAQs (Frequently Asked Questions)
- How can machine learning benefit small businesses?
- What are the limitations of machine learning?
- How can businesses address bias in machine learning algorithms?
- Are there any industries that still need to embrace machine learning fully?
- How can businesses ensure the privacy and security of data in machine learning applications?
Machine learning refers to the ability of computer systems to learn and improve from experience without explicit programming. It involves the development of algorithms that can analyse data, identify patterns, and make predictions or decisions. In a business context, machine learning algorithms can extract valuable insights from large datasets and give businesses a competitive edge.
1. The Impact of Machine Learning on Business
Machine learning has had a transformative impact on businesses across various sectors. Here are some key areas where machine learning is driving significant changes:
– Enhanced decision-making processes
Machine learning algorithms enable businesses to make data-driven decisions based on accurate and timely insights. By analysing vast amounts of structured and unstructured data, these algorithms can identify trends, patterns, and anomalies humans might overlook. This helps businesses optimise their decision-making processes and stay ahead of the competition.
– Improved customer experience
Machine learning is a game-changer when it comes to improving customer experience. Businesses can personalise their offerings and provide targeted recommendations by analysing customer data, such as purchase history, browsing behaviour, and social media interactions. This level of personalisation enhances customer satisfaction, increases engagement, and boosts loyalty.
– Streamlined operations and cost reduction
Machine learning can optimise various operational aspects of a business, leading to cost reductions and increased efficiency. For example, predictive maintenance algorithms can analyse sensor data to identify potential equipment failures before they occur, allowing businesses to schedule maintenance proactively. This minimises downtime, reduces repair costs, and improves overall operational efficiency.
2. How can you use machine learning in your business?
Sales and Marketing industry
Sales and marketing departments can benefit immensely from machine learning techniques. Here’s how machine learning is transforming these areas:
– Personalised recommendations and targeted marketing
Machine learning algorithms can analyse customer behaviour, preferences, and historical data to provide personalised recommendations and targeted marketing campaigns. Businesses can deliver relevant content and offers by understanding individual customer needs and preferences, significantly increasing conversion rates and sales.
– Predictive analytics for sales forecasting
Machine learning models can analyse historical sales data, market trends, and external factors to predict future sales more accurately. This enables businesses to make informed decisions about inventory management, production planning, and resource allocation, ultimately improving profitability.
– Customer segmentation and behaviour analysis
Machine learning algorithms can segment customers based on their attributes, behaviours, and preferences. This segmentation helps businesses tailor their marketing strategies and communication to specific customer groups, resulting in more effective campaigns and higher customer satisfaction.
Example of a successful business that utilises machine learning.
Netflix is a global streaming platform that offers subscribers a wide range of movies, TV shows, and original content. The platform leverages machine learning algorithms to analyse user data and provide personalised recommendations to each individual user.
Netflix collects vast amounts of data on user viewing habits, such as the movies and TV shows they watch, the genres they prefer, and the time of day they engage with the platform. Machine learning algorithms analyse this data to understand user preferences and patterns. By identifying similarities between users with similar viewing histories and preferences, Netflix can generate accurate recommendations for new content.
The recommendation system powered by machine learning is crucial in enhancing the user experience on Netflix. It helps users discover new content that aligns with their interests and preferences, increasing engagement and customer satisfaction. Additionally, the personalised recommendations contribute to longer user retention and reduced churn rate for Netflix.
Machine learning also enables Netflix to optimise its content production and acquisition strategies. By analysing user data and identifying popular content genres, Netflix can make data-driven decisions about which shows and movies to produce or license. This approach minimises the risk of investing in content that may not resonate with the audience, ensuring a higher likelihood of success.
Finance and Risk Assessment industry
The finance industry heavily relies on data analysis and risk assessment. Machine learning has made significant strides in this sector by enabling:
– Fraud detection and prevention
Machine learning algorithms can detect patterns indicative of fraudulent activities, such as suspicious transactions or abnormal behaviour. By continuously monitoring financial data, these algorithms can identify potential fraud cases in real-time, saving businesses from financial losses.
– Credit scoring and loan approval
Machine learning models can analyse credit history, income data, and other relevant factors to assess the creditworthiness of individuals or businesses. This automated credit scoring process helps lenders make informed decisions about loan approvals, reducing risks and improving the efficiency of lending operations.
– Portfolio management and investment strategies
Machine learning algorithms can analyse vast amounts of financial data and market trends to identify investment opportunities and optimise portfolio management strategies. These algorithms can process information at a scale and speed that surpasses human capabilities, assisting asset managers in making data-driven investment decisions.
Example of a successful business that utilises machine learning.
Ant Financial, the financial arm of Alibaba Group, is a leading provider of financial services and leverages machine learning algorithms to analyse extensive financial data. They employ machine learning to enhance accuracy, efficiency, and scalability.
One key application of machine learning at Ant Financial is credit scoring and loan approval. By analysing factors such as credit history, income data, and transaction patterns, machine learning models assess the creditworthiness of individuals and businesses. This automated credit scoring process streamlines lending operations, reduces risks, and enables efficient service for a large customer base.
Machine learning algorithms also play a crucial role in fraud detection and prevention within Ant Financial’s financial ecosystem. The algorithms enable immediate action to prevent fraud, protect customers, and minimise financial losses by continuously monitoring transaction data and identifying patterns and anomalies indicative of fraudulent behaviour.
Ant Financial employs machine learning algorithms in investment strategies and portfolio management to analyse market data, financial indicators, and customer preferences. These algorithms process vast amounts of data quickly, identify investment opportunities, and make data-driven decisions to maximise returns while managing risks effectively.
Additionally, machine learning is utilised in risk assessment and pricing models for various financial products. These models accurately assess risks associated with loans, insurance policies, and investment products by analysing historical data, market trends, and external factors. This enables Ant Financial to determine appropriate pricing, manage risk exposure, and ensure sustainable profitability.
Automation and Efficiency industry
Machine learning has the potential to automate and optimise various business processes. Here are some examples of how it is driving automation and efficiency:
– Process automation and optimisation
By leveraging machine learning, businesses can automate repetitive and time-consuming tasks, freeing up human resources for more strategic activities. Intelligent automation powered by machine learning can improve efficiency, accuracy, and scalability across departments, contributing to productivity gains.
– Supply chain management
Machine learning algorithms can analyse historical supply chain data, external factors, and demand patterns to optimise inventory management, production planning, and logistics. This helps businesses minimise stockouts, reduce inventory holding costs, and improve supply chain efficiency.
– Quality control and predictive maintenance
Machine learning algorithms can analyse sensor data from manufacturing processes to identify anomalies and predict equipment failures. By detecting potential issues in real-time, businesses can take proactive measures to maintain product quality, reduce downtime, and enhance customer satisfaction.
Example of a successful business that utilises machine learning.
UiPath is a leading Robotic Process Automation (RPA) platform that enables businesses to automate repetitive tasks and streamline workflows using software robots.
A few key applications of machine learning in UiPath’s operations:
Intelligent document processing: Machine learning algorithms extract valuable information from unstructured documents such as invoices, purchase orders, and contracts. UiPath’s software robots can accurately extract data and automate document processing tasks by analysing document patterns and using natural language processing techniques, reducing manual effort and increasing efficiency.
Process discovery and optimisation: UiPath’s machine learning algorithms can analyse user interactions with software applications and identify repetitive tasks or process bottlenecks. This enables businesses to discover areas for automation and process optimisation, improving efficiency and reducing costs.
Cognitive automation: Machine learning enables software robots to understand and process unstructured data such as text, images, and videos. This cognitive automation capability allows UiPath’s robots to perform complex tasks that require understanding and decision-making, such as sentiment analysis, image recognition, and data classification.
Predictive analytics: UiPath incorporates machine learning algorithms to perform predictive analytics on historical data. The algorithms can predict future outcomes by analysing past trends and patterns, helping businesses make data-driven decisions and optimise their operations.
Healthcare and Medicine Industry
The healthcare industry has embraced machine learning to revolutionise patient care, diagnostics, and drug development. Here’s how it is transforming healthcare:
– Disease diagnosis and treatment planning
Machine learning algorithms can analyse patient data, medical records, and diagnostic images to assist healthcare professionals in disease diagnosis and treatment planning. These algorithms help improve diagnostic accuracy, optimise treatment plans, and enhance patient outcomes by providing accurate and timely insights.
– Drug discovery and development
Machine learning plays a crucial role in accelerating drug discovery and development processes. By analysing large datasets and molecular structures, machine learning algorithms can identify potential drug candidates, predict their efficacy, and optimise their molecular properties. This significantly speeds up the drug development pipeline and contributes to discovering more effective treatments.
– Health monitoring and predictive analytics
Machine learning algorithms can analyse patient-generated health data, wearable devices, and electronic health records to monitor health conditions, detect early signs of deterioration, and predict disease progression. This proactive approach enables healthcare providers to intervene on time, leading to better patient management and improved healthcare outcomes.
Example of a successful business that utilises machine learning.
IBM’s Watson Health Watson Health is a division of IBM that focuses on applying artificial intelligence and machine learning technologies to healthcare-related challenges.
Watson Health leverages machine learning algorithms to analyse and interpret vast amounts of medical data, including patient records, medical literature, research studies, and imaging data. Here are a few critical applications of machine learning in Watson Health’s operations:
Medical image analysis: Machine learning algorithms analyse medical images, such as X-rays, MRIs, and CT scans. Watson Health’s machine learning models can help radiologists and other healthcare professionals detect abnormalities, identify patterns, and make more accurate diagnoses.
Clinical decision support: Watson Health’s machine learning capabilities enable it to analyse and interpret patient data from electronic health records (EHRs), medical journals, and other sources. By processing this data, Watson Health can provide evidence-based treatment recommendations, alert healthcare professionals to potential drug interactions or allergies, and assist in personalised treatment planning.
Drug discovery and development: Watson Health utilises machine learning algorithms to analyse biomedical research, clinical trials, and drug databases. By analysing vast amounts of data, Watson Health can identify potential drug targets, predict drug efficacy, and aid in the discovery of new treatments and therapies.
Population health management: Watson Health applies machine learning to analyse population health data, including demographics, disease prevalence, and social determinants of health. This allows healthcare providers and policymakers to identify at-risk populations, predict disease outbreaks, and allocate resources more effectively.
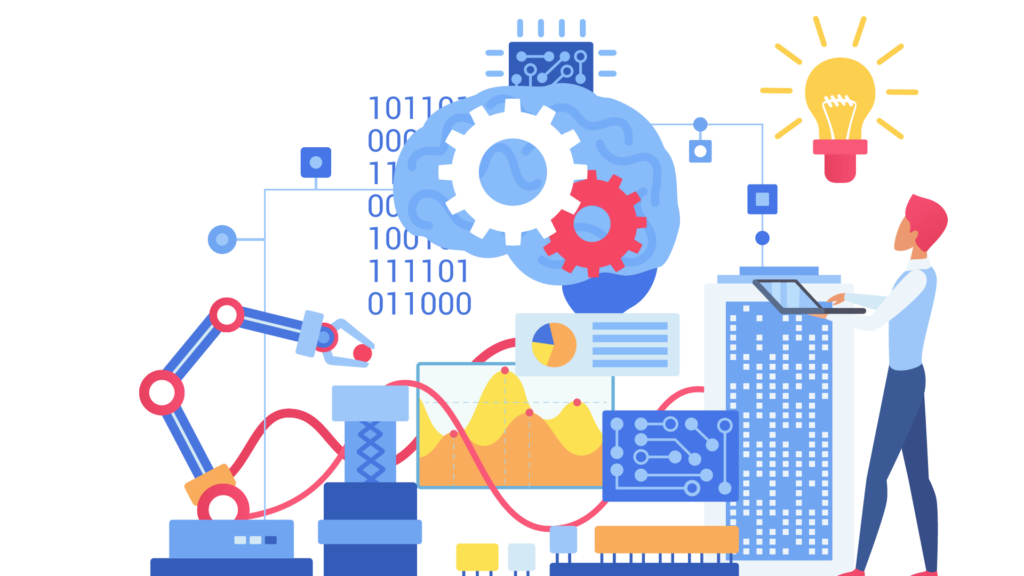
3. The Future of Machine Learning in Business
The future of machine learning in business looks promising, with several advancements on the horizon. Here are some key areas to watch out for:
– Advancements in natural language processing
Natural language processing (NLP) is an area of machine learning focused on enabling computers to understand and interact with human language. As NLP technologies advance, businesses can expect significant improvements in customer support chatbots, sentiment analysis, and language translation.
– Integration of machine learning with the Internet of Things (IoT)
Integrating machine learning with the Internet of Things (IoT) opens up new data analysis and automation possibilities. By leveraging machine learning algorithms on IoT devices, businesses can process data at the edge, reduce latency, and make real-time decisions. This integration can revolutionise smart homes, autonomous vehicles, and industrial automation.
– Potential for autonomous systems and robotics
Machine learning is a key enabler for autonomous systems and robotics. As algorithms become more sophisticated and capable, businesses can expect advancements in areas such as autonomous vehicles, drones, and robotic process automation. These technologies have the potential to reshape industries, enhance productivity, and improve safety.
Machine learning has become an indispensable tool for businesses, allowing them to harness the power of data and make informed decisions. From improving customer experience to streamlining operations, the impact of machine learning is pervasive across various sectors. However, as businesses embrace machine learning, addressing ethical considerations, ensuring transparency, and prioritising data privacy is essential. With advancements in natural language processing, integration with the Internet of Things, and the potential for autonomous systems, the future of machine learning in business is bright.
4. FAQs (Frequently Asked Questions)
- How can machine learning benefit small businesses?
Machine learning can benefit small businesses by improving operational efficiency, enhancing customer experience, and enabling data-driven decision-making. It can automate tasks, personalise marketing efforts, and provide insights that help businesses optimise their operations and compete effectively.
- What are the limitations of machine learning?
Machine learning algorithms rely on historical data to make predictions or decisions, which means they may struggle with new or unseen situations. They can also be sensitive to biased or incomplete data, leading to inaccurate results. Additionally, ethical concerns and the need for interpretability pose challenges for some machine learning applications.
- How can businesses address bias in machine learning algorithms?
To address bias in machine learning algorithms, businesses should ensure diverse and representative datasets, regularly monitor and audit algorithmic outputs, and implement fairness-aware techniques. It is important to have a multidisciplinary team that includes experts in ethics, diversity, and algorithmic fairness.
- Are there any industries that still need to embrace machine learning fully?
While machine learning has made significant inroads in many industries, some sectors are still in the early stages of adopting these technologies. Industries such as agriculture, construction, and hospitality have the potential to benefit from machine learning. Still, they may require further exploration and customisation for their specific needs.
- How can businesses ensure the privacy and security of data in machine learning applications?
To ensure the privacy and security of data in machine learning applications, businesses should implement robust data protection measures, including encryption, access controls, and secure data storage. They should comply with relevant data protection regulations and regularly assess and update their security protocols to address emerging threats.
Very good knowledge